AI is much hyped but often misunderstood
Artificial intelligence is reaching the top of its ‘hype cycle’, but the term is often misused leaving marketers at risk of handing over cash for solutions that do nothing new and offer little value.
Artificial intelligence continues to garner much attention from marketers eager to learn how it can make their lives easier. Of the 12 stages at the Festival of Marketing 2017, for example, the AI stage was perhaps where audiences were most rapt. Marketers were dutifully listening to industry experts and wondering whether off-the-shelf machine learning solutions exist to match their needs, or indeed if such solutions are everything their vendors say they are.
Marketers now have the daunting task of navigating the ‘AI summer’, a term given to the current period of excitement about the technology.
Previous summers have come and gone. The term ‘machine learning’ was coined in 1959, and Nicola Millard of BT Global Services says she has been working on it for the last 27 years. But the current AI summer is significant because it comes along when marketing seems ready for it.
We may not be close to ‘strong AI’, where artificial intelligence matches that of humans, but the flood of ‘big data’, faster processors and open source algorithms that kick-started this summer are all grist to the mill of new marketing channels and software.
Machine learning as ‘automation-plus’
So, how can marketers avoid the hype, and being stung for ‘AI’ tech or platforms which deliver little?
We’ve already seen many brands scramble to launch chatbots, most of which may, at best, serve a specific customer need, but at worst are gimmicky decision trees with little capability in natural language processing.
READ MORE: How Adidas, Just Eat and HTC are using chatbots
Steffan Aquarone, consultant head of best practice reports at Econsultancy, gave some helpful advice to marketers at the Festival of Marketing. First off, he encouraged the audience to think of machine learning as “automation-plus”.
“In reality, that’s what a lot of it is – but with a lot more decision-making capability than before.”
Aquarone gave a number of use cases but one that struck a chord was from Travelodge. The hotel chain has experimented in differentiating between business and leisure travellers using large-scale interrogation of real-time data.
Previously, an assumption would be made based on the channel a customer used or their last recorded booking. But this method was massively misleading for a key segment – people booking their own travel for business and leisure. No one person booking on the Travelodge website is necessarily restricted to any one segment.
Machine learning is helping Travelodge to profile the intention of each customer at each visit. This analysis can look at data such as last booking, frequency of types of booking, search details, stay duration, ancillaries booked, device, time of day, etc.
The ability to perform this analysis in real time means that independent business travellers are no longer lumped into the ‘wrong’ segment for a particular booking.
This kind of example is a good one for machine learning newbies to consider – it’s a step up from automation as it involves prediction, and makes use of systems and data streams that are often already cloud based.
In this way, machine learning becomes a layer applied to your marketing stack, as shown in the diagram below from Econsultancy blogger and CRM expert Chris O’Hara.
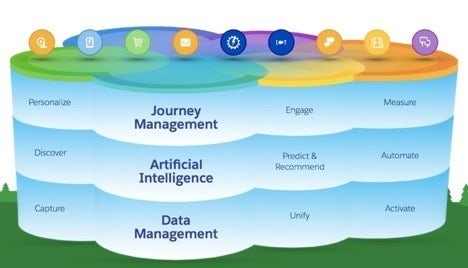
Tips for working with machine learning suppliers
There are many more examples like Travelodge – prosaic but incredibly useful and representing sound marketing thinking.
Aquarone called on marketers to remain level-headed when looking for machine learning tech suppliers, giving the following tips:
- Go beyond the pretty slides and ask questions about how a supplier’s technology works.
- Look at the questions a supplier asks – if they just want all your data to begin with, they are likely planning to use it to develop their solution, rather than having it ready or investigating your needs.
- Look for microservices that can slot in on top of your existing marketing stack.
- Avoid long deployments – you should be able to start testing the technology pretty quickly.
- Look for the ability to learn as you go and to be able to write back to systems.
The machine learning hype is much like the mobile hype of five to 10 years ago. Aquarone made the analogy of companies that simply wanted an app, with agencies only too happy to build them one without the proper level of thinking being applied.
An appetite for innovation?
While Aquarone’s advice is much needed to cut through the hype, there is room for an addendum here.
Large organisations are already committing to building their own machine learning capability, even if fleshing out a data science team and “a few neural networks” doesn’t make what computer science professor Andrew Ng would call an AI company.
And there’s also an opportunity for smaller businesses. Jim Sterne, author of ‘Artificial Intelligence for Marketing: Practical Applications’, recently told me that one question marketers need to ask themselves is “What is the tolerance in my organisation for testing and innovation?”
READ MORE: How brands are using artificial intelligence to enhance customer experience
Some small and nimble companies may well look to partner with emerging startups in this area and forge a mutually beneficial relationship. Sterne highlights the case of Cosabella, a family-owned lingerie company in the US that is working with an AI platform called Albert.
He says the brand acts “like Albert’s product development team”, getting to experiment with the technology, while Albert benefits from technical improvements that will deliver value for other clients. This type of partnership may not be for every organisation, but the results for Cosabella have been positive and widely reported.
Sterne says AI is reaching the top of its ‘hype cycle’, and is the “most misused” term today, however: “There’s a reason for the hype in AI. Whether it’s voice recognition, natural language processing, computer vision, or robotics – all are incredibly useful. But what marketers have to understand is machine learning.”
And crucially, he adds, “you have to understand it well enough to understand how you might put it to work”.
Ben Davis is editor at Marketing Week’s sister title Econsultancy.